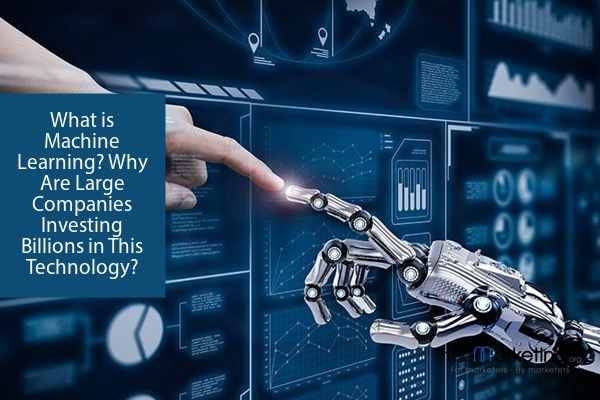
What is Machine Learning? Why Are Large Companies Investing Billions in This Technology?
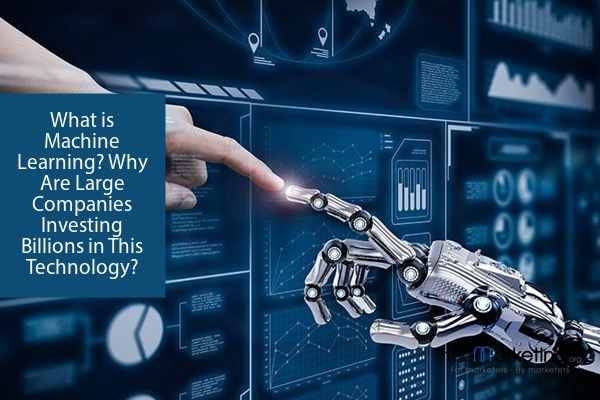
Discover the benefits and applications of machine learning, and why large companies like Google, Facebook, Amazon, Microsoft, and IBM are investing billions in this technology. Explore the challenges of implementation and the future of machine learning, including advancements in deep learning, IoT integration.
Machine learning is a rapidly growing technology that has gained the attention of many large companies in recent years. This innovative technology involves the use of algorithms and statistical models to enable computer systems to learn from data and improve their performance over time. In this blog post, we will explore what machine learning is, why it is so valuable, and how it is being used by some of the world's biggest tech companies. Additionally, we will examine the challenges that come with implementing machine learning and the exciting advancements that lie ahead for this cutting-edge technology. So, let's dive in and discover the world of machine learning.
1. What is Machine Learning?
Machine Learning is a subset of artificial intelligence that involves the use of algorithms and statistical models to enable computer systems to improve their performance on a specific task over time, without being explicitly programmed to do so. It involves the training of a machine to learn and make predictions or decisions based on data patterns, rather than relying on explicit instructions. This technology has the potential to transform the way businesses operate, by automating processes, improving decision-making, and enhancing personalization. In the following sections, we will explore the benefits, applications, challenges, and future of Machine Learning, as well as the major companies investing in this technology.
2. Benefits of Machine Learning
Machine Learning has become an increasingly popular technology in recent years due to its numerous benefits. Firstly, it enables increased efficiency by automating repetitive tasks and reducing the need for human intervention. Secondly, it leads to improved decision-making by providing insights and predictions based on data analysis. Thirdly, it offers enhanced personalization by tailoring experiences to individual users, resulting in higher customer satisfaction. Lastly, it reduces costs by streamlining processes and minimizing errors. These benefits have made Machine Learning a highly sought-after technology in various industries, ranging from healthcare to finance to retail.
Increased Efficiency
Machine Learning has been a buzz phrase in the technology industry for a while now, and for good reason. One of the major benefits of Machine Learning is its ability to increase efficiency in various processes. By automating certain tasks that would otherwise require human intervention, Machine Learning can save time and resources for companies. For example, in the healthcare industry, Machine Learning algorithms can help with the interpretation of medical images, reducing the time it takes for doctors to make a diagnosis. In the finance industry, Machine Learning models can assist with fraud detection, helping financial institutions to prevent financial losses. By allowing machines to handle repetitive and time-consuming tasks, companies can focus on more important aspects of their business.
Improved Decision-Making
Machine learning is transforming the way organizations make decisions. By analyzing vast amounts of data and identifying patterns, machine learning algorithms can provide valuable insights into business operations, customer behavior, and market trends. This, in turn, enables companies to make better, data-driven decisions. With the help of machine learning, businesses can quickly analyze large datasets, identify trends, and make predictions based on the patterns detected. This helps businesses to identify potential opportunities and risks, and make informed decisions about how to proceed.
One of the most significant benefits of machine learning for decision-making is the ability to automate decision-making processes. By automating routine decisions, organizations can save time and reduce errors. Automated decision-making can also help organizations to make more consistent decisions across different departments and locations, ensuring that they are always operating in the most efficient way possible. Additionally, machine learning can help organizations to identify and mitigate risks before they become major issues. By analyzing data in real-time, machine learning algorithms can detect anomalies and identify patterns that may indicate potential risks.
Overall, machine learning has the potential to revolutionize the way businesses approach decision-making. By providing insights that would otherwise be difficult or impossible to obtain, machine learning can help organizations to make more informed, data-driven decisions. This, in turn, can lead to increased efficiency, reduced costs, and improved performance across all areas of the organization.
Enhanced Personalization
Machine learning has revolutionized the way businesses interact with their customers by enabling enhanced personalization. By analyzing data from various sources, including social media, search history, and purchase behavior, machine learning algorithms can predict customer preferences and provide tailored recommendations. This personalized approach can improve customer satisfaction and loyalty, ultimately leading to increased revenue for businesses. Additionally, machine learning can help companies track customer behavior over time, enabling them to identify trends and adjust their strategies accordingly.
One example of enhanced personalization through machine learning is the use of chatbots in customer service. These bots can use natural language processing to understand customer inquiries and provide personalized responses in real-time. This technology can significantly reduce response times, improving customer satisfaction.
Another application of machine learning for enhanced personalization is in the healthcare industry. By analyzing patient data, medical professionals can develop personalized treatment plans based on individual characteristics and medical history. This approach can improve treatment outcomes and reduce healthcare costs by identifying potential health issues early on.
Overall, enhanced personalization through machine learning has the potential to transform the way businesses interact with their customers and improve outcomes in various industries. As technology continues to advance, we can expect to see even more personalized experiences for consumers, from targeted advertising to individualized healthcare.
Reduced Costs
Machine learning has proven to be a valuable tool for reducing costs across a variety of industries. By automating tasks and improving efficiency, companies can save money on labor expenses and decrease the likelihood of costly errors. Additionally, machine learning can help identify areas where waste can be reduced and optimize processes to maximize profitability. In this section, we will explore some of the ways in which machine learning is being used to reduce costs.
One of the primary ways in which machine learning is reducing costs is by automating repetitive tasks that would normally require human intervention. For example, in the manufacturing industry, machine learning algorithms can be used to monitor production lines and identify potential issues before they become major problems. This can lead to significant cost savings by reducing the need for manual inspections and minimizing downtime.
Another way in which machine learning is reducing costs is by improving supply chain efficiency. By analyzing data on inventory levels, shipping times, and other factors, machine learning algorithms can help companies optimize their supply chain processes to reduce costs and improve delivery times. This can be particularly valuable for companies that operate in highly competitive industries where even small improvements in efficiency can lead to significant cost savings.
Finally, machine learning is being used to reduce costs in the healthcare industry by improving patient outcomes and reducing the need for expensive interventions. For example, machine learning algorithms can be used to predict which patients are most likely to require readmission to the hospital, allowing healthcare providers to intervene early and avoid costly hospital stays. Additionally, machine learning can be used to identify patients who are at high risk for developing chronic conditions, allowing for early intervention and improved outcomes.
Overall, the ability of machine learning to automate tasks, optimize processes, and improve outcomes makes it a powerful tool for reducing costs across a variety of industries. While there are certainly challenges to implementing machine learning effectively, the potential cost savings and other benefits make it an attractive investment for many companies.
3. Applications of Machine Learning
Machine learning has an extensive range of applications across various industries. In image and speech recognition, machine learning algorithms can accurately identify and classify objects, faces, and voices. This technology is widely used in the entertainment industry, security systems, and automotive industry. Fraud detection is another area where machine learning has proven to be highly effective. Financial institutions and e-commerce businesses use machine learning algorithms to analyze transactions and identify fraudulent activities. Predictive maintenance is another application of machine learning, which uses data analytics techniques to predict equipment failures and schedule maintenance before a breakdown occurs. Recommendation systems, which provide personalized recommendations to customers, are also powered by machine learning algorithms. These systems are commonly used in e-commerce, entertainment, and social media platforms.
Image and Speech Recognition
Image and Speech Recognition: Machine learning has enabled the development of systems that can recognize and interpret images and speech with increasing accuracy. Image recognition systems are used in a variety of applications, such as self-driving cars, security surveillance, and healthcare diagnostics. Speech recognition systems, on the other hand, are used in virtual assistants, customer service, and language translation. These applications are made possible by the ability of machine learning algorithms to analyze large amounts of data and identify patterns that can be used to make accurate predictions and decisions.
Fraud Detection
Fraudulent activities are a major concern for both individuals and businesses. Machine learning has proven to be a powerful tool in detecting fraud in various industries such as finance, insurance, and e-commerce. By analyzing large amounts of data, machine learning algorithms can identify patterns and anomalies that indicate fraudulent behavior. This has led to a significant reduction in financial losses due to fraud.
One of the main benefits of machine learning in fraud detection is its ability to adapt to new fraud patterns. Traditional rule-based systems can only detect fraud based on pre-defined rules and are not able to identify new types of fraudulent activities. In contrast, machine learning algorithms can continuously learn from new data and adjust their detection methods accordingly. This makes them more effective in detecting both known and unknown fraud patterns.
Another advantage of machine learning in fraud detection is its ability to analyze large amounts of data in real-time. This allows for faster detection of fraudulent activities and reduces the likelihood of financial losses. Machine learning algorithms can also identify false positives, which are cases where legitimate transactions are mistakenly flagged as fraudulent. This helps to reduce the number of unnecessary investigations and improves the overall efficiency of fraud detection systems.
Overall, machine learning has revolutionized fraud detection by providing more accurate and efficient methods for identifying fraudulent activities. As businesses continue to face new and evolving fraud threats, machine learning will play an increasingly important role in protecting against financial losses and maintaining customer trust.
Predictive Maintenance
Predictive maintenance is another important application of machine learning that has gained the attention of many industries, including manufacturing, transportation, and energy. Predictive maintenance involves using machine learning algorithms to analyze data from sensors and other sources to predict when equipment is likely to fail, allowing companies to perform maintenance before the failure occurs. This approach can significantly reduce downtime, increase equipment lifespan, and reduce maintenance costs.
One example of predictive maintenance is General Electric's Predix platform, which uses machine learning to predict when turbines, locomotives, and other equipment are likely to fail. The platform analyzes data from sensors, maintenance records, and other sources to identify patterns and predict when maintenance is needed. This approach has helped GE reduce downtime, increase equipment lifespan, and save millions of dollars in maintenance costs.
Another example of predictive maintenance is the use of machine learning to predict when vehicles are likely to fail. This approach can help transportation companies schedule maintenance more efficiently, reducing downtime and improving safety. For example, UPS uses machine learning to predict when its trucks are likely to break down, allowing the company to schedule maintenance before a breakdown occurs.
Overall, predictive maintenance is an important application of machine learning that can help companies reduce costs, increase efficiency, and improve safety. As the technology continues to advance, we can expect to see more companies adopting predictive maintenance solutions to optimize their operations.
Recommendation Systems
Recommendation systems are an important application of machine learning that have gained significant popularity in recent years. These systems are designed to provide personalized recommendations to users based on their past behavior, preferences, and interests. They are widely used by e-commerce platforms, streaming services, and social media sites to suggest products, movies, and content that users are likely to find interesting.
One of the main benefits of recommendation systems is that they improve customer engagement and satisfaction. By providing users with personalized recommendations, these systems can help them discover new products and services that they might not have found otherwise. This, in turn, can increase sales and revenue for businesses.
There are several types of recommendation systems, including content-based, collaborative filtering, and hybrid systems. Content-based systems analyze the attributes of items and recommend similar items to users. Collaborative filtering systems, on the other hand, use the behavior of similar users to make recommendations. Hybrid systems combine both approaches to provide more accurate and diverse recommendations.
Despite their benefits, recommendation systems also face several challenges. These include the cold start problem, where new users or items have no data available for recommendations, and the sparsity problem, where there is insufficient data for accurate recommendations. Additionally, there are ethical concerns around the use of recommendation systems, particularly regarding privacy and the potential for reinforcing biases.
Overall, recommendation systems are an important application of machine learning that have revolutionized the way businesses interact with their customers. As the amount of data available continues to grow, these systems are likely to become even more accurate and effective, providing users with even more personalized and relevant recommendations.
4. Large Companies Investing in Machine Learning
Large companies across various industries are heavily investing in machine learning technology. One of the main reasons for this is the potential for significant business benefits, such as increased efficiency, improved decision-making, enhanced personalization, and reduced costs. Some of the largest players in the tech industry, including Google, Facebook, Amazon, Microsoft, and IBM, have invested billions of dollars in machine learning research and development.
Google, for example, has been using machine learning algorithms for years to improve its search results, speech recognition, and image recognition. The company has also invested in deep learning research, which involves training neural networks with large amounts of data to improve accuracy in tasks such as object recognition and natural language processing.
Facebook has also been heavily investing in machine learning, particularly in the area of natural language processing. The company has developed a machine learning-powered chatbot that can understand and respond to natural language queries, and it is also using machine learning algorithms to personalize users' news feeds and improve ad targeting.
Amazon is another company that has been investing heavily in machine learning, particularly in the area of recommendation systems. The company uses machine learning algorithms to analyze customers' purchase histories and browsing behavior to make personalized product recommendations.
Microsoft has also been heavily investing in machine learning, particularly in the area of speech recognition. The company has developed a machine learning-powered speech recognition system that can transcribe spoken words with high accuracy, even in noisy environments.
IBM has been investing in machine learning for a wide range of applications, including fraud detection, predictive maintenance, and personalized marketing. The company has also developed its own machine learning platform, IBM Watson, which is used in industries such as healthcare and finance.
Overall, large companies are investing in machine learning technology because of its potential for significant business benefits. However, there are also challenges to implementing machine learning, such as data quality and quantity, a lack of skilled professionals, ethical concerns, and integration with existing systems. Despite these challenges, the future of machine learning looks promising, with advancements in deep learning, increased integration with IoT, expansion of autonomous systems, and growing importance in healthcare and finance industries.
Google is one of the largest and most prominent companies investing in machine learning. The search giant has been at the forefront of artificial intelligence (AI) research for years, and has made significant strides in applying machine learning to its products and services. Google's machine learning projects span a wide range of applications, from image and speech recognition to natural language processing and predictive analytics.
One of the most well-known examples of Google's machine learning capabilities is its search algorithm. Google uses machine learning algorithms to understand user queries and provide more relevant search results. The company also uses machine learning to improve its advertising platform, allowing advertisers to target specific audiences more effectively.
Another area where Google has invested heavily in machine learning is in its Google Assistant product. Google Assistant uses machine learning algorithms to understand natural language queries and provide intelligent responses. The company has also integrated machine learning into its Google Translate service, allowing users to translate text and speech in real-time.
In addition to its consumer-facing products, Google is also investing in machine learning for enterprise applications. The company's Cloud AutoML platform allows businesses to build custom machine learning models without requiring extensive expertise in data science or machine learning. Google is also using machine learning to improve its cybersecurity offerings, using algorithms to detect and prevent cyberattacks before they occur.
Overall, Google's investment in machine learning is driven by its belief that AI and machine learning will play a central role in the future of technology. The company is committed to developing innovative products and services that leverage these technologies to improve efficiency, decision-making, and personalization for its users.
Facebook is another large company that heavily invests in machine learning. The social media giant uses this technology to personalize the user experience by providing tailored content and advertisements based on user data. In addition, Facebook employs machine learning algorithms to detect and remove fake accounts and content that violates community standards. The company also uses image and speech recognition to automatically tag photos and videos uploaded by users. With over 2.7 billion monthly active users, Facebook collects a vast amount of data that can be utilized to further improve its machine learning capabilities.
Amazon
Amazon is one of the largest companies in the world that has made a significant investment in machine learning. The company has been using machine learning algorithms to improve its e-commerce platform and provide a personalized shopping experience to its customers. With the help of machine learning, Amazon can analyze customer data to understand their preferences and behavior, and recommend products accordingly. This has led to a significant increase in customer satisfaction and sales for the company.
Moreover, Amazon is also using machine learning for fraud detection and prevention. The company has been able to detect and prevent fraudulent activities on its platform by analyzing user behavior and transaction data. This has helped Amazon to protect its customers and maintain trust in its platform.
In addition, Amazon is using machine learning for predictive maintenance of its fulfillment centers. The company uses data from various sensors installed in its warehouses to predict when a component is likely to fail and replace it before it causes any downtime. This has helped Amazon to ensure smooth operations and minimize downtime due to maintenance issues.
Amazon has also developed a number of machine learning-based products and services such as Amazon Web Services (AWS) and Alexa. AWS provides a range of cloud-based machine learning tools and services that can be used by businesses to build and deploy machine learning models. Alexa, on the other hand, is an AI-powered virtual assistant that uses natural language processing and machine learning to understand and respond to user queries.
Despite its success in machine learning, Amazon faces several challenges in implementing the technology. One of the biggest challenges is the quality and quantity of data required for training machine learning models. Amazon needs to collect and store large volumes of data to build accurate models, which can be a costly and time-consuming process.
Another challenge is the lack of skilled professionals who can develop and deploy machine learning models. Amazon needs to hire data scientists, machine learning engineers, and other professionals with expertise in the field, which is a highly competitive market.
Overall, Amazon's investment in machine learning has helped the company to improve its operations, provide better customer experiences, and develop innovative products and services. As machine learning continues to advance, it is likely that Amazon will continue to be at the forefront of the technology's development and application.
Microsoft
Microsoft is another large company investing heavily in machine learning. The tech giant has been integrating machine learning into its products and services, such as Office 365 and the Azure cloud platform, to help businesses make more informed decisions. One of Microsoft's key machine learning tools is Azure Machine Learning, which enables developers to build, train, and deploy machine learning models in a cloud-based environment.
Microsoft has also been exploring the use of machine learning in various industries, including healthcare and finance. In healthcare, the company has developed machine learning models to help doctors diagnose diseases more accurately and efficiently. In finance, Microsoft is using machine learning to identify fraudulent activities and improve risk management.
However, like other companies investing in machine learning, Microsoft faces challenges in implementing the technology. One of the biggest challenges is ensuring the quality and quantity of data used to train machine learning models. Microsoft is addressing this challenge by partnering with industry leaders in data collection and analysis.
Another challenge is the lack of skilled professionals in the field of machine learning. To address this, Microsoft has launched the Microsoft Professional Program for Artificial Intelligence, which provides training and certification in machine learning and other AI-related fields.
As machine learning continues to evolve, Microsoft is at the forefront of advancing the technology. The company is exploring advancements in deep learning, which involves training models with large datasets to improve accuracy and efficiency. Additionally, Microsoft is integrating machine learning with the Internet of Things (IoT) to enable smarter devices and systems.
Overall, Microsoft's investment in machine learning is a testament to the potential of the technology in improving business operations and transforming industries.
IBM
IBM has been investing heavily in machine learning technology for several years now. In 2014, the company launched Watson, an AI platform that uses natural language processing and machine learning to analyze vast amounts of data and provide insights to businesses. Since then, IBM has been working on expanding Watson's capabilities and integrating it into various industries.
One of IBM's major focuses has been on healthcare. The company has partnered with several healthcare organizations to develop AI-powered tools that can help doctors diagnose diseases faster and more accurately. For example, Watson for Oncology uses machine learning to analyze patient data and suggest personalized cancer treatment plans. IBM has also developed AI-powered medical imaging tools that can detect diseases like breast cancer and diabetic retinopathy.
Another area where IBM has been investing in machine learning is finance. The company has developed AI-powered financial risk management tools that can analyze vast amounts of financial data and provide real-time insights to traders. IBM has also partnered with several banks to develop chatbots that can provide customer support and help customers manage their finances.
Overall, IBM's investments in machine learning are driven by the belief that AI can help businesses and organizations make faster and more informed decisions. The company is continuing to expand Watson's capabilities and is exploring new applications for machine learning across a range of industries.
5. Challenges of Implementing Machine Learning
Implementing machine learning is not without its challenges. One of the biggest hurdles is ensuring the quality and quantity of data used in the algorithms. Without sufficient and relevant data, machine learning models can produce inaccurate or biased results. This requires companies to invest in data infrastructure and management, which can be costly and time-consuming.
Another challenge is the shortage of skilled professionals who can develop and implement machine learning systems. The demand for data scientists and machine learning engineers is high, but the supply is limited. This has led to a talent gap that companies are struggling to fill, especially in industries where machine learning is critical to success.
Ethical concerns are also a challenge in implementing machine learning. There have been instances where algorithms have produced discriminatory results or violated privacy laws. Companies need to ensure that their models are transparent and fair, and that they are not misusing personal data or infringing on individuals' rights.
Integration with existing systems is another challenge. Companies need to ensure that their machine learning systems are compatible with their current infrastructure and software. This requires careful planning and coordination with IT departments and other stakeholders.
Overall, while the benefits of machine learning are significant, it is not a plug-and-play technology. Companies need to overcome these challenges to successfully implement machine learning in their operations.
Data Quality and Quantity
One of the biggest challenges in implementing machine learning is ensuring data quality and quantity. Machine learning algorithms rely heavily on large sets of data to train and improve their accuracy. However, this data must be accurate, relevant, and representative of the problem the algorithm is trying to solve. Poor quality data can lead to biased or inaccurate results, which can have significant consequences in fields such as healthcare and finance.
In addition to data quality, the quantity of data available can also be a challenge. In some cases, companies may not have access to enough data to train their algorithms effectively. This can be especially true in industries such as healthcare, where data privacy regulations can limit the amount of data that can be collected and shared. As a result, companies may need to invest in data collection and storage infrastructure to support their machine learning initiatives.
Another challenge related to data is the need for ongoing data maintenance and updates. As data sources change or new types of data become available, machine learning algorithms may need to be retrained or updated to stay accurate and relevant. This requires ongoing investment in data management and processing capabilities.
Overall, ensuring data quality and quantity is a critical component of successful machine learning implementations. Companies must invest in robust data management infrastructure and processes to support their machine learning initiatives and ensure accurate, unbiased results.
Lack of Skilled Professionals
The adoption of machine learning technologies is heavily dependent on a skilled workforce capable of developing, implementing, and maintaining these systems. However, there is a significant shortage of professionals with the necessary expertise to work in this emerging field. According to a report by LinkedIn, the demand for machine learning engineers has grown by 9.8 times since 2012, making it the fastest-growing job category in the United States. This shortage of skilled professionals is a major challenge for companies looking to invest in machine learning.
One reason for the shortage of skilled professionals is the lack of formal education and training programs in this field. Many universities have only recently started offering courses and degrees in machine learning, and the number of graduates with expertise in this area is still limited. Another reason is the high demand for these professionals, which has led to intense competition for talent and inflated salaries.
The lack of diversity in the field is another concern. Women and people from underrepresented groups are significantly underrepresented in the machine learning workforce. A study by the AI Now Institute found that only 18% of authors at leading AI conferences were women. This lack of diversity can lead to biased algorithms and models, which can have serious consequences in areas such as criminal justice, hiring, and lending.
To address the shortage of skilled professionals, companies are investing in various initiatives, such as training programs, partnerships with universities, and recruitment efforts. Some companies are also developing tools and platforms that make it easier for non-experts to use machine learning technologies. These efforts are crucial to ensuring that the benefits of machine learning are accessible to a wider range of businesses and industries.
Ethical Concerns
As the use of machine learning continues to grow, there are also growing ethical concerns surrounding the technology. One major concern is the potential for bias in algorithms, particularly in areas such as hiring and lending decisions. This bias can be compounded by the use of historical data that reflects past discrimination and perpetuates it in the present. Additionally, the use of machine learning in surveillance and monitoring raises concerns about privacy and individual rights. The ability of machines to continuously learn and adapt also raises questions about accountability and transparency, as it can be difficult to trace the decision-making process of complex algorithms. As machine learning becomes more integrated into our daily lives, it is important to address these ethical concerns and ensure that the technology is used in a responsible and equitable manner.
Integration with Existing Systems
Integration with Existing Systems
One of the biggest challenges in implementing machine learning is integrating it with existing systems. Many companies have legacy systems and databases that are not compatible with the advanced algorithms used in machine learning. This can result in a complex and time-consuming process of data extraction, transformation, and loading. In addition, integrating machine learning with existing systems requires a thorough understanding of the business processes and requirements.
To overcome these challenges, companies need to invest in modernizing their IT infrastructure to support machine learning. This can involve upgrading databases, implementing cloud-based solutions, and providing access to real-time data. In addition, companies need to ensure that their IT teams have the necessary skills to integrate machine learning with existing systems. This includes knowledge of programming languages such as Python, R, and Java, as well as experience with big data processing and analysis.
Another challenge of integrating machine learning with existing systems is ensuring data security and privacy. Machine learning relies on large amounts of data, which can be sensitive and confidential. Companies need to implement robust security measures to protect this data from unauthorized access and ensure compliance with regulations such as GDPR and HIPAA.
Overall, integrating machine learning with existing systems requires a strategic approach and a significant investment of time and resources. However, the benefits of machine learning, such as improved efficiency and decision-making, make it a worthwhile investment for companies looking to stay competitive in today's data-driven world.
6. Future of Machine Learning
As machine learning continues to evolve and mature, there are several exciting advancements on the horizon that promise to revolutionize the way we interact with technology. One area of particular interest is deep learning, which involves training artificial neural networks to recognize patterns and make decisions based on complex data sets. This approach has already shown great promise in areas like natural language processing and computer vision, and is expected to continue to improve in accuracy and efficiency as more data becomes available.
Another key trend to watch is the integration of machine learning with the Internet of Things (IoT), which will enable devices to collect and analyze vast amounts of data in real-time. This will lead to a proliferation of smart, connected systems that are able to make intelligent decisions and take actions autonomously, without human intervention.
In addition to these technical advancements, machine learning is also poised to play an increasingly important role in key industries like healthcare and finance. In healthcare, machine learning algorithms are being used to analyze patient data and develop more personalized treatment plans, while in finance, they are being used to detect fraud and improve risk management.
Of course, as with any new technology, there are also challenges to be addressed as machine learning continues to evolve. These include ethical concerns around the use of personal data, as well as the need for skilled professionals who can design and implement effective machine learning systems.
Despite these challenges, the future of machine learning looks bright, with the potential to transform a wide range of industries and improve our lives in countless ways. As more companies invest in this technology and more data becomes available, we can expect to see even more exciting advancements in the years to come.
Advancements in Deep Learning
Advancements in Deep Learning have been a major driver in the growth of Machine Learning technology. Deep Learning refers to the use of neural networks with multiple layers to analyze and interpret complex data. The deeper the neural network, the more complex the data it can handle. Recent advancements in Deep Learning have led to breakthroughs in areas such as image and speech recognition, natural language processing, and autonomous systems.
One of the key advancements in Deep Learning has been the development of convolutional neural networks (CNNs). CNNs are a type of neural network that is particularly effective at analyzing images and video. They work by breaking down an image into smaller parts, analyzing each part individually, and then combining the results to form a complete understanding of the image. This has led to significant improvements in image recognition technology, with applications in areas such as self-driving cars and medical imaging.
Another important area of advancement in Deep Learning is natural language processing (NLP). NLP refers to the ability of machines to understand and interpret human language. This includes tasks such as language translation, sentiment analysis, and chatbots. Recent breakthroughs in NLP have been driven by the development of transformer models, such as BERT and GPT-3. These models use attention mechanisms to analyze the context of words within a sentence, leading to more accurate language processing.
Overall, advancements in Deep Learning have been a major driver in the growth of Machine Learning technology. As these technologies continue to evolve, we can expect to see even more breakthroughs in areas such as autonomous systems, healthcare, and finance.
Increased Integration with IoT
With the increasing popularity of the Internet of Things (IoT), machine learning has found a new avenue for growth and development. The integration of machine learning with IoT has the potential to transform the way we interact with technology and how technology interacts with us. Machine learning algorithms can help IoT devices to learn from their environment and adapt to changing conditions, making them more efficient and effective. This integration has already been seen in various industries such as manufacturing, transportation, and healthcare, where IoT sensors are being used to gather data and machine learning algorithms are being used to analyze that data in real-time.
One of the key benefits of integrating machine learning with IoT is the ability to perform predictive maintenance. By analyzing data from sensors on machines and equipment, machine learning algorithms can identify patterns and predict when maintenance is needed. This can help to reduce downtime, improve efficiency, and lower maintenance costs. Machine learning can also be used to improve energy efficiency in buildings by analyzing data from IoT sensors and adjusting heating, cooling, and lighting systems accordingly.
Another area where machine learning and IoT are being integrated is in the development of autonomous systems. Autonomous vehicles, drones, and robots are becoming increasingly common, and machine learning is playing a crucial role in their development. Machine learning algorithms can help these systems to learn from their environment and adapt to changing conditions, making them more effective and efficient.
As the number of IoT devices continues to grow, the amount of data being generated is also increasing exponentially. This presents a challenge for machine learning, as it requires large amounts of high-quality data to train algorithms effectively. However, advances in edge computing and distributed machine learning are helping to address this challenge by enabling data to be processed and analyzed at the source, rather than being sent to a central location for analysis.
Overall, the integration of machine learning with IoT has the potential to revolutionize the way we interact with technology and how technology interacts with us. As more industries adopt this technology, we can expect to see increased efficiency, improved decision-making, and enhanced personalization in a wide range of applications.
Expansion of Autonomous Systems
As machine learning continues to advance, there is a growing trend towards the expansion of autonomous systems. Autonomous systems are those that can operate and make decisions without human intervention. This technology is being used in a variety of industries, including transportation, manufacturing, and healthcare. In the transportation industry, for example, autonomous vehicles are being developed that can drive themselves without the need for a human driver. In manufacturing, autonomous robots are being used to perform tasks such as assembling products or packaging items. In healthcare, autonomous systems are being developed to help diagnose and treat patients, as well as to monitor their health remotely.
One of the key benefits of autonomous systems is that they can help improve efficiency and productivity. By eliminating the need for human intervention, these systems can operate around the clock without the need for breaks or rest periods. They can also perform tasks faster and more accurately than humans, which can help reduce errors and improve quality.
However, there are also challenges associated with the development and implementation of autonomous systems. One of the biggest challenges is ensuring that these systems are safe and reliable. This requires extensive testing and validation to ensure that the systems can operate safely in a variety of conditions and scenarios.
Another challenge is ensuring that autonomous systems are secure and protected against cyber-attacks. As these systems become more connected to the internet and other networks, they become vulnerable to hacking and other security threats. It is important to develop robust security measures to protect these systems and the data they generate.
Despite these challenges, the expansion of autonomous systems is expected to continue in the coming years. As machine learning and other technologies continue to advance, we are likely to see more and more autonomous systems being developed and implemented in a wide range of industries and applications.
Growing Importance in Healthcare and Finance Industries
Machine learning has become an increasingly important technology in various industries, including healthcare and finance. In healthcare, machine learning algorithms can analyze medical data to provide accurate diagnoses, identify potential health risks, and improve patient outcomes. For example, machine learning models can predict the likelihood of readmission or complications after surgery, enabling healthcare providers to take preventive measures. In finance, machine learning can help banks and financial institutions detect fraud, predict market trends, and identify investment opportunities.
Moreover, machine learning can also improve the efficiency of healthcare and finance operations. For instance, machine learning algorithms can automate administrative tasks, such as data entry and claims processing, reducing the workload of healthcare and finance professionals. This, in turn, can lead to reduced costs and increased productivity.
In healthcare, machine learning can also enable personalized treatment plans based on an individual's medical history, genetic data, and lifestyle. This can help healthcare providers tailor treatment to the specific needs of each patient, resulting in better outcomes and fewer adverse events.
In the finance industry, machine learning can improve customer experience by providing personalized investment advice and tailored financial products. For example, machine learning algorithms can analyze customer data to identify their investment goals, risk tolerance, and financial situation, and offer customized investment portfolios.
Overall, the growing importance of machine learning in healthcare and finance industries is set to revolutionize the way these sectors operate, leading to improved outcomes, reduced costs, and better customer experiences.
Summary
In conclusion, machine learning is a rapidly evolving technology that has the potential to transform various industries. Large companies are investing billions in this technology because it can help them make more informed decisions, improve customer experiences, and drive business growth. Machine learning algorithms can analyze vast amounts of data and identify patterns that humans may not be able to detect, which can lead to more accurate predictions and insights. As the technology continues to advance, we can expect to see even more applications of machine learning in the future. Whether you're a business owner or a technology enthusiast, it's important to stay informed about this exciting field and the potential it holds.