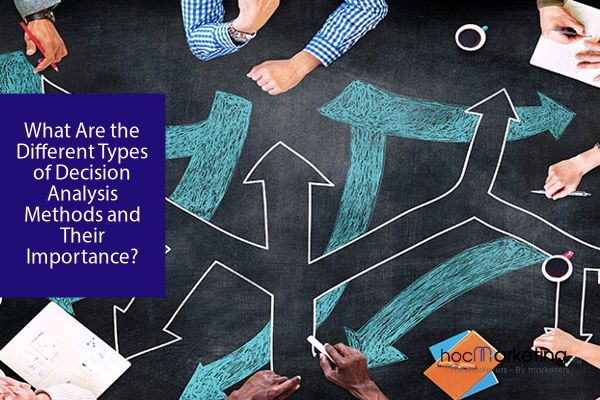
What Are the Different Types of Decision Analysis Methods and Their Importance?
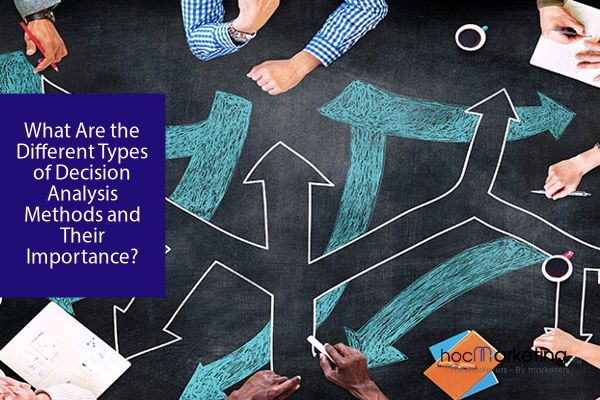
Decision analysis is a structured approach to problem-solving that involves identifying and assessing contributing factors It helps in selecting the best solution and implementing it effectively Its importance lies in making informed decisions and achieving optimal outcomes Methods include decision trees, expected value, Bayesian networks, Monte Carlo simulation, multicriteria decision analysis, utility theory, linear programming, and heuristics Real-world examples demonstrate its effectiveness
By utilizing a structured approach to decision-making, decision analysis (DA) involves a comprehensive evaluation of the factors contributing to a problem or opportunity. This then leads to the development and assessment of potential solutions, making it a valuable tool for decision-makers. DA can be applied to making decisions about single courses of action or comparing multiple options, enabling consideration of all relevant factors in identifying the optimal solution.
What is Decision Analysis (DA)?
Decision analysis (DA) is a systematic approach used to assess the potential results of various options and select the most optimal one based on the available resources and constraints. The aim of DA is to identify the best solution to a problem or opportunity.
Understanding Decision Analysis
involves the use of quantitative, visual, and systematic techniques to make crucial business decisions. It utilizes a variety of tools, such as psychology, economic principles, and management theories to formally assess important aspects of a problem and develop an optimal treatment strategy. Ultimately, decision analysis is a powerful tool that can aid in making informed and effective decisions.
The methodology of decision analysis is utilized to analyze all relevant data, incorporating elements of psychology, management, training, and economics, to assist with the decision-making process. This approach is commonly adopted for decisions that require an evaluation of multiple variables and potential outcomes or goals. Whether it's for risk management, capital investment, or strategic business decisions, individuals or teams can benefit from this approach.
The primary objective of decision analysis is to ensure that decisions are made after considering all pertinent information and alternatives. This approach can be beneficial for companies making million-dollar investment decisions or individuals planning their retirement savings. The principles of decision analysis can be applied to a wide range of issues, from complex business situations to everyday matters.
History of Decision Analysis (da)
Subjective probability was first introduced by mathematical philosopher Frank Ramsey in 1931 to represent an individual's beliefs or uncertainties. In the 1940s, mathematician John von Neumann and economist Oskar Morgenstern developed utility theory as a way to express preferences over uncertain scenarios. Later, statistician Leonard Jimmie Savage developed an alternative axiomatic structure for decision analysis in the early 1950s, resulting in expected-utility theory based on a complete axiomatic structure for making decisions under uncertain situations.
The early breakthroughs in decision analysis were later refined and made accessible to a wider audience, resulting in the creation of a range of decision analysis techniques that are now commonly taught in business schools and industrial engineering departments. One such introductory text was published by Howard Raiffa of Harvard Business School in 1968, which was followed by further developments in utility theory by Ralph Keeney and Howard Raiffa in 1976. In 1977, a set of readings on decision analysis by Ron Howard of Stanford University and Jim Matheson was published, which was later expanded into a two-volume work in 1984. The Decision Analysis Society was established as a special interest group within the Operations Research Society of America (ORSA) in 1980, which later merged with The Institute of Management Sciences (TIMS) to form the Institute for Operations Research and Management Sciences (INFORMS). Since 2004, INFORMS has published Decision Analysis as a specialized journal.
How Decision Analysis Works?
The decision analysis (da) process is divided into five distinct steps
1. Defining the problem or opportunity
This step involves identifying the factors that contribute to a problem or opportunity.
2. Identifying the objectives
This step involves identifying the goals that the decision-maker wants to achieve.
3. Developing possible solutions
This step involves developing and evaluating possible solutions to the problem or opportunity.
4. Selecting the best solution
This step involves choosing the best possible solution, based on the objectives and constraints.
5. Implementing the solution
The next crucial step is putting the chosen solution into action and closely monitoring its progress. Decision analysis (DA) is a powerful tool for decision-makers seeking to weigh all pertinent information and factors when assessing risks or opportunities and determining the optimal course of action. While it's important to acknowledge that DA isn't foolproof and won't always lead to the best outcome, it's an invaluable aid in making informed decisions.
Importance of Decision Analysis Process
The significance of the decision analysis process lies in its ability to take into account all pertinent information when making decisions. This process is also instrumental in identifying the optimal solution, taking into consideration the limitations and resources available.
Leveraging decision analysis can prove to be highly advantageous for businesses and organizations, as it aids in enhancing the quality of decisions made. Not only does it reduce the associated risks, it also assists in saving valuable resources such as time and money. This powerful tool can be applied across multiple scenarios, enabling businesses to make sound decisions based on reliable data.
Making investment decisions
Choosing a marketing strategy
Selecting a new product or service
Developing a business plan
Making organizational changes
Evaluating risks and opportunities
When to Use Decision Analysis
Decision analysis can be used in a variety of situations, including:
When there are multiple objectives that need to be considered
When there are multiple options available
When there is uncertainty about the future
When resources are limited
When the consequences of a decision are significant
If you find yourself in one of these situations, then DA may be the right tool for you.
Decision Analysis Methods
There are a variety of methods that can be used in decision analysis. Some of the most common methods include:
- Decision trees
- Utility theory
- Simulation
- Linear programming
- Decision matrices
- Heuristics, etc
Which method you use will depend on the situation you are in, and the type of decision you need to make.
Decision Trees
A decision tree is an excellent visual aid that represents the decision-making process graphically. It provides a clear visualization of the various steps involved in making a decision. Decision trees are versatile and can be used for multiple purposes, such as:
Understanding the different steps involved in making a decision
Determining which factors are most important in making a decision
Evaluating different options
Analyzing risks and opportunities
Expected Value (EV)
Businesses and organizations can benefit greatly from the use of decision trees, as they have the potential to enhance decision-making quality. Proper utilization of decision trees can mitigate decision-making risks, while simultaneously saving valuable resources such as time and money.
The formula for calculating expected value involves determining the probability of each potential outcome and multiplying it by the value of that outcome. This calculation provides a statistical measure of the expected outcome of a decision.
The expected value formula is a useful tool to determine the potential outcome of a decision. It involves calculating the sum of all possible events, taking into account their respective probabilities and values. This allows for a more informed decision-making process by providing insight into the potential outcomes.
Let's say you're faced with a decision between investing in stock A or stock B. Stock A has a 50/50 chance of either increasing in value by 10% or decreasing in value by 5%. Meanwhile, stock B has a 60/40 chance of either increasing in value by 15% or decreasing in value by 10%.
Stock A: (0.5 x 0.1) + (0.5 x -0.05) = 0.025
Stock B: (0.6 x 0.15) + (0.4 x -0.1) = 0.045
In this example, the expected value of stock B is higher than the expected value of stock A. This means that, on average, stock B is a better investment than stock A.
Bayesian Networks
A Bayesian network serves as a visual aid for decision analysis, presenting a graphical representation of the decision-making process. It is a useful tool for simplifying the complex steps involved in making a decision. The applications of a Bayesian network are diverse, ranging from gaining a better understanding of decision-making steps to facilitating decision-making processes.
Determining which factors are most important in making a decision
Evaluating different options
By utilizing Bayesian networks, businesses and organizations can effectively analyze risks and identify potential opportunities. This powerful tool can ultimately enhance the decision-making process, leading to more informed and successful outcomes. In doing so, businesses can mitigate potential risks and capitalize on advantageous opportunities, ultimately leading to increased efficiency and profitability.
Monte Carlo Simulation
Monte Carlo simulation is a powerful statistical tool that allows us to generate numerous possible outcomes based on various input parameters. This technique is particularly useful in situations where there is a high degree of uncertainty surrounding the future events and outcomes. By running multiple simulations, Monte Carlo simulation can help us identify the most likely scenarios and inform our decision-making process.
In the scenario of deciding whether to invest in stock A, one may face uncertainty regarding future outcomes. However, Monte Carlo simulation can be implemented to predict potential results by modeling various possibilities. This simulation provides valuable insight into the probable outcome of one's investment decision.
Multicriteria decision analysis (MCDA)
In order to evaluate multiple options, the technique of multicriteria decision analysis (MCDA) is commonly employed. It proves to be useful when there are various factors that need to be taken into consideration, and when comparing options using a single criterion becomes challenging.
When faced with the decision of investing in either stock A or stock B, it's important to take into account a variety of factors such as potential return, level of risk, and associated costs. To make an informed decision, MCDA can be utilized to evaluate each option based on the specific criteria chosen. With the help of MCDA, it becomes easier to determine which stock is most likely to yield a successful outcome.
Utility Theory
Utility theory aims to measure the level of satisfaction an individual derives from a specific decision. To evaluate the worth of various options, multi-attribute utility theory comes into play, facilitating the comparison of their respective utilities.
Linear Programming
Linear programming is a powerful mathematical tool that enables the optimization of a linear function. It is particularly useful when there are multiple variables to consider, and finding the optimal solution using a single criterion is challenging. One example of such a scenario is resource allocation. With limited funds and time, linear programming can help identify the best possible way to allocate resources while adhering to pre-defined constraints.
Heuristics
Simple rules called heuristics can aid in decision-making, particularly in situations where there is ambiguity and comparing options based on a single criterion is challenging.
A heuristic is a mental shortcut that allows you to make a quick decision based on limited information. When faced with a complex decision, like choosing between two stocks, heuristics can be helpful in simplifying the process and making a decision within a limited amount of time. One common heuristic is to choose the stock with the higher expected return, which can help you quickly narrow down your options and make a decision.
Decision Analysis Modeling
Decision analysis modeling is a powerful tool in management science that can offer significant benefits in a range of scenarios. One such model that is frequently employed is the influence diagram, which helps to illustrate the connections between various factors in a problem. By deconstructing a problem into its individual parts and analyzing the relationships between them, decision analysis can facilitate the decision-making process for intricate situations.
Decision Analysis Real World Examples
Some of the common examples of the use of decision analysis methods are
In a business strategic decisions group, to study customer buying behavior or to decide on a new product launch
In healthcare, to choose the most effective treatment for a patient
In government, to develop public policy
In marketing multi-attribute decision making, to identify target markets or design promotional campaigns
In manufacturing, to select the most efficient production process
In logistics, to develop distribution plans or choose transportation routes.
Conclusion
Using Decision Analysis as a tool for evaluating multiple options can lead to better decision-making by considering various variables and criteria. Its effectiveness in providing clarity about uncertain outcomes cannot be denied.
What are some examples of when Decision Analysis could be used to find favorable final outcomes? Please share your thoughts in the comments section below.